This work investigates the uncertainty in 3D pose estimation of DenseFusion, a Deep Learning-based 3D Object Pose Estimation (3DOPE) framework. The accuracy and precision of object spatial localization is evaluated on the benchmark Yale Carnegie Berkeley-Video Dataset. The Average Euclidean Distance metric is employed as a figure of merit for the quantitative data analysis. Moreover, the Average Rotational and Translational Distances among the estimated and the ground-truth poses are analysed. Results gathered on the YCB- Video Dataset are validated on a newly collected 3DOPE dataset, the ISS Dataset. The latter is populated by harnessing a 3D scanner, an RGB-D camera, and an anthropomorphic robot. Outcomes highlight an Average Euclidean Distance value of 1.8 mm on the best-processed item in the YCB-Video Dataset. Similarly, an Average Euclidean Distance value of 1.9 mm is achieved on the ISS Dataset. Eventually, the measurement uncertainty of the six components of the 3D poses is inspected. The latter assessment is performed on the ISS Dataset by relying on the ISO GUM [1].
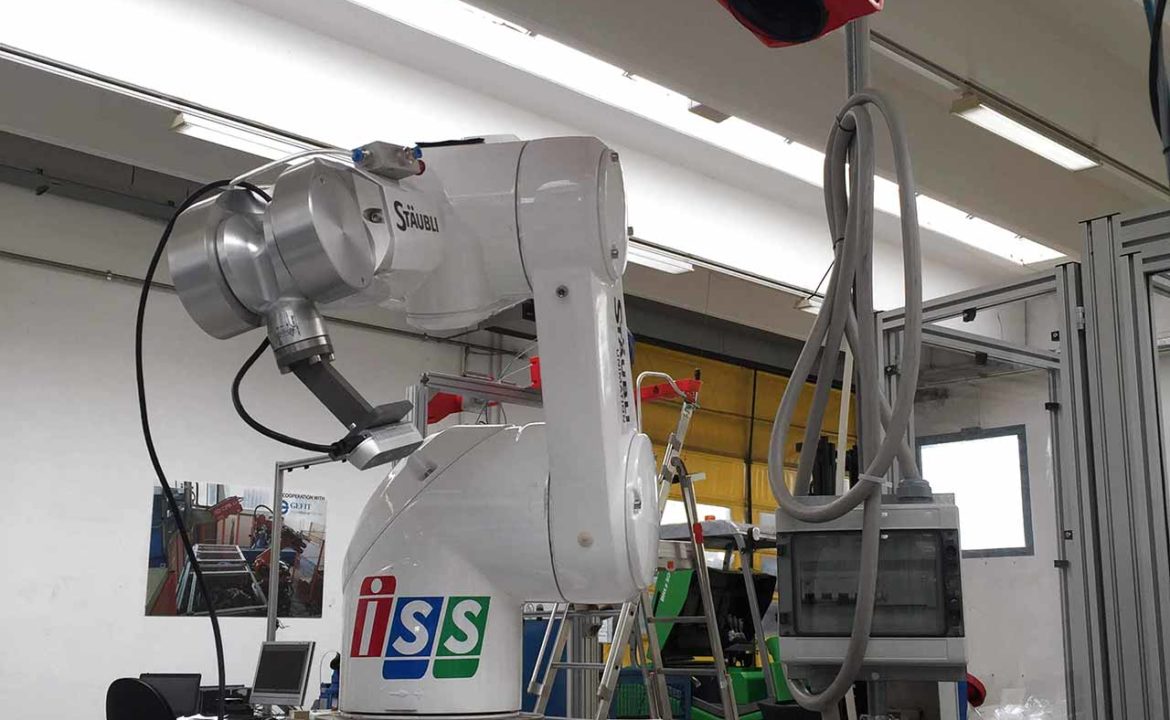